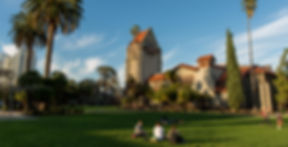
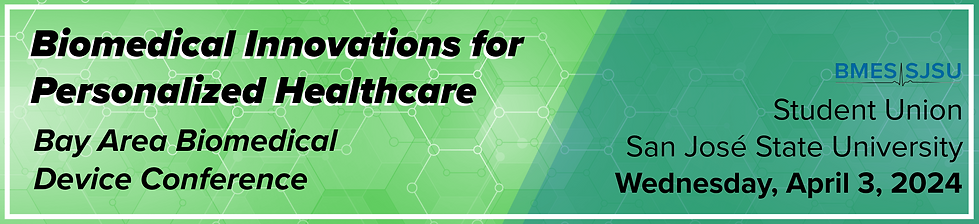
Machine Learning for Studying Neural Degeneration in Botryllus Schlosseri over Time
Oz Shachef - Biomedical Engineering Department, SJSU;
Conor Morris - Biomedical Engineering Department, SJSU
Dr. Abdulmelik Mohammed and Dr. Ayelet Voskoboynik
Technical Advisor:
Aging is the primary risk factor for most neurodegenerative diseases, which affected more than 7 million American patients in 2023. In an effort to better understand and design treatment for neurodegenerative diseases, a quantifiable statistic is needed for the effects that aging has on the degeneration of the brain, neural stem cells, and the associated nervous system. Botryllus schlosseri is a marine invertebrate chordate that shares approximately 75% sequence homology between its protein-encoding genes with human genes [1]. Two neurodegeneration pathways characterize the life of Botryllus - a cyclic pathway represented by the weekly replacement of the zooids and a continuous one as the colony ages. During both the weekly degeneration cycles and colony aging, the brain undergoes dramatic changes in the differential expression of 200 genes with mammalian homology associated with human neurodegenerative diseases, including those whose genetic variations can predict early onset of neurodegeneration [2]. Botryllus, with its accelerated cell replacement cycle, makes a great model for predicting effects in humans, which we leverage for our research. Our work involves creating a way to automatically analyze the video data provided by Stanford’s Voskoboynik lab and extract specific biological markers representative of the health of the organism’s brain/neural network using a computer vision machine learning model. Here we will be demonstrating our model's ability to classify, segment, and measure data from the videos, as we work to understand the effects of aging on neural health and translate those results to humans in further research.